Despite significant progress in 3D deep learning for tasks such as classification
and semantic segmentation, robust change detection techniques
for complex, coloured environments have not been developed.
This is in part due to the absence of labelled change detection datasets
and the inherent difficulty of constructing such datasets despite the
abundance of unlabeled data. Flow Compare is a fully unsupervised
approach that leverages expressive generative models with iterative
attention trained on multi-temporal coloured point clouds. Change
detection is achieved by reframing the problem as anomaly detection
given a learnt conditional distribution. Training pairs are formed
by co-registered multi-temporal extracts from coloured point cloud
scenes. The inherent class imbalance due to the rarity of semantically
important change, which is problematic for supervised approaches,
is here harnessed to guarantee that relevant changes are considered
anomalies under the learnt distribution. This approach shows promise
in detecting not only geometric change but also colour change whilst
being robust to common semantically unimportant change.
Image
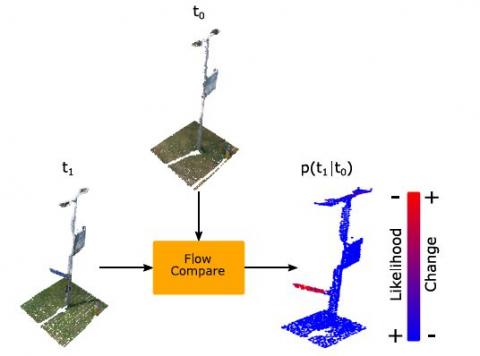